Why Retrieval-Augmented Generation is the Next Big Thing in AI
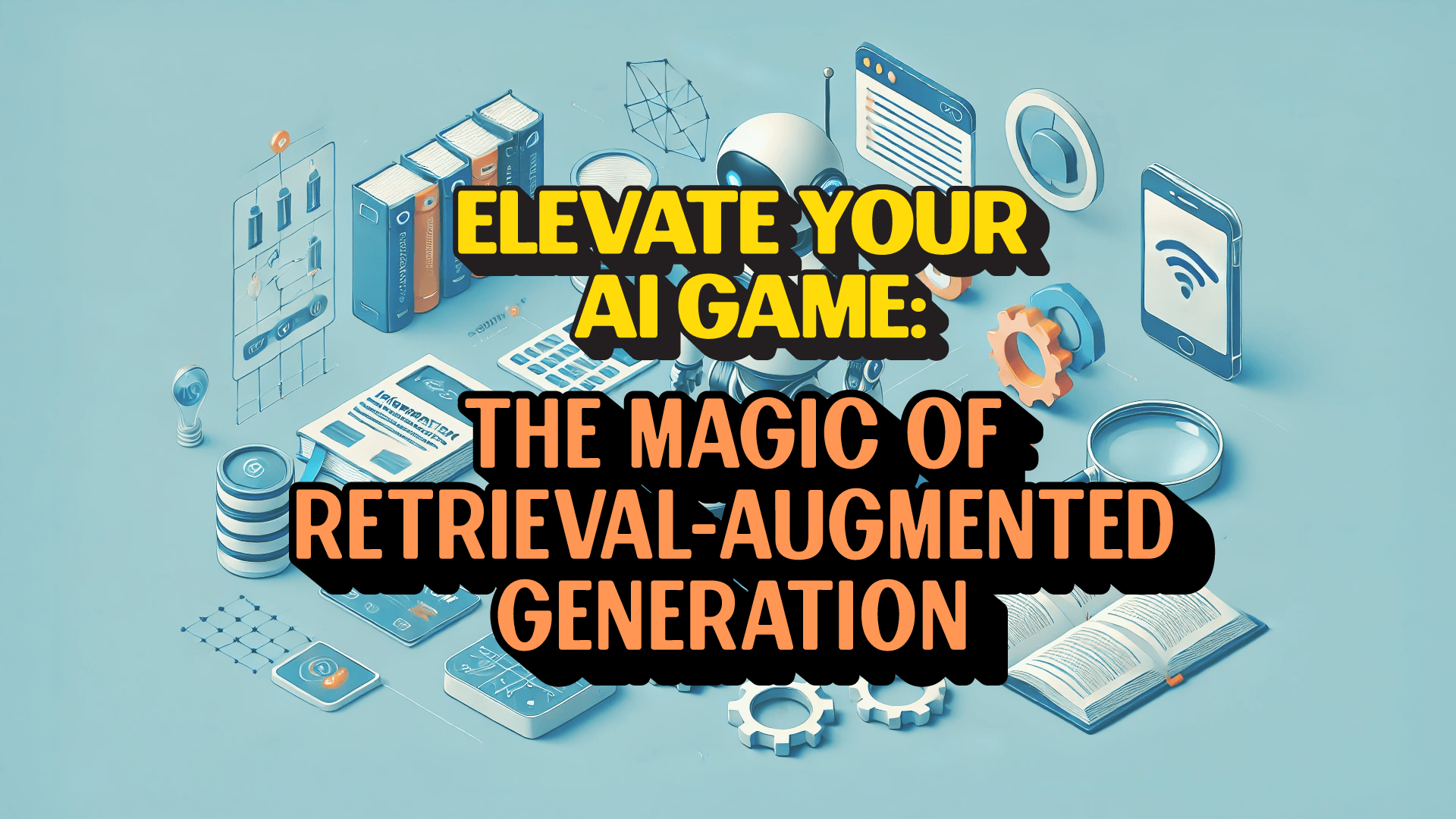
Ever wish an AI could pull in the latest information when giving you an answer? That’s where Retrieval-Augmented Generation (RAG) comes in. It’s like having a super-smart assistant that knows when to look things up before answering you.
Breaking It Down
RAG is pretty clever. It combines two things:
-
Retriever: This part is like a supercharged search engine. When you ask a question, it digs through a knowledge base (think databases, documents, or even the web) to find relevant information. It’s almost like asking a librarian to grab the books you need before you start writing.
-
Generator: Once the retriever has done its job, the generator (usually a language model like GPT-4) steps in. It uses both the info you provided and what the retriever found to give a more complete and up-to-date response.
Why Bother?
The big win with RAG is that it can fill in gaps where a regular AI model might be outdated or missing info. It’s especially useful for niche topics or situations where you need the latest facts. Instead of relying solely on its training data, the AI “knows” when to fetch more details, making its responses more relevant and accurate.
How Could a Startup Use This?
Let’s say you’re building a platform that helps businesses stay on top of market trends. Your clients rely on you to keep them informed about the latest shifts in their industry. Using RAG, your platform could automatically pull in the most recent news, research, and relevant stats from various sources. When clients ask for insights, the system quickly gathers the latest information, providing them with up-to-date, personalized reports. This way, your team can spend more time refining the product rather than manually tracking down data.
As a founding engineer at a startup, I find RAG an exciting way to automate data gathering, keeping things current without extra manual effort.
What’s Next?
This is just a sprout. I plan to expand on RAG’s use cases and how it stacks up against other AI approaches. Stay tuned!